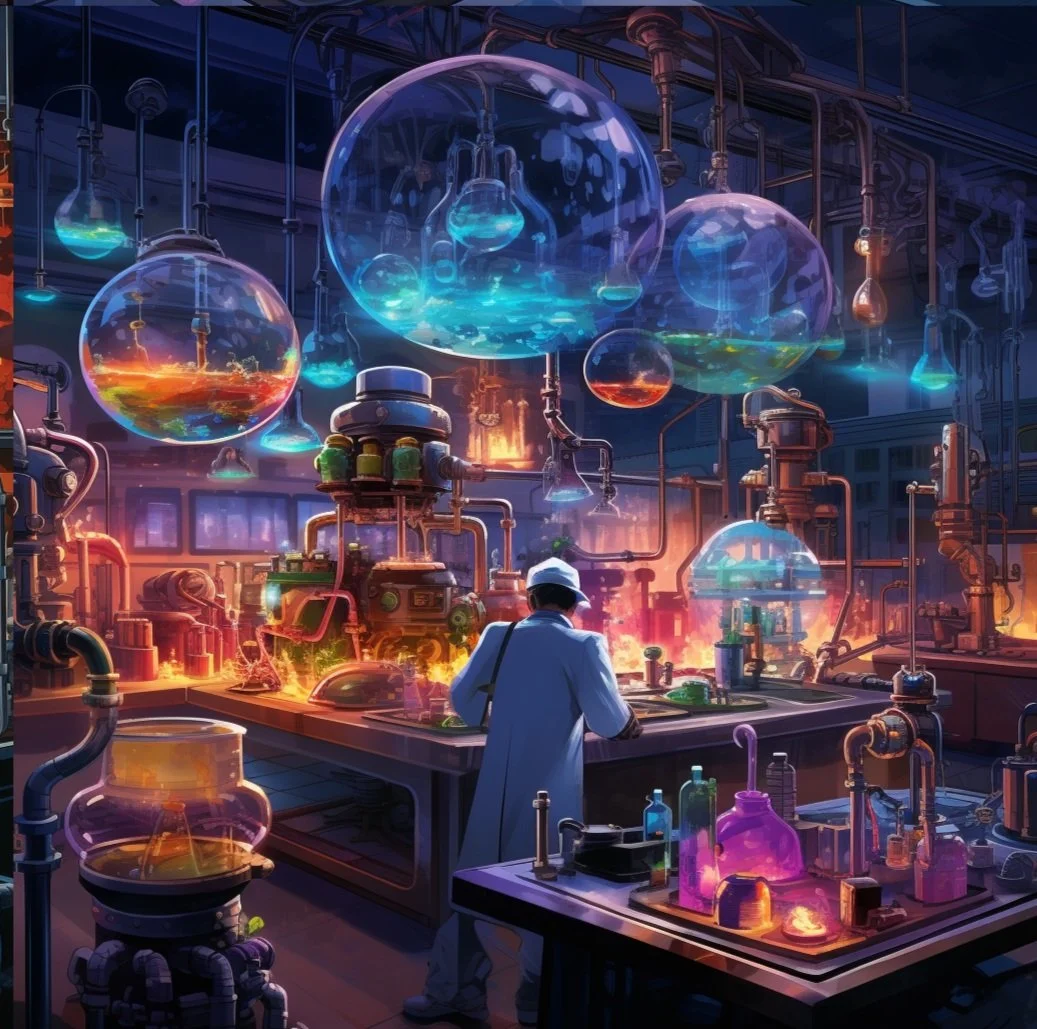
Research
Our research is at the exciting intersection of materials science, robotics, and artificial intelligence. We encourage creative approaches that seamlessly weave high-tech into scientific processes, poised to tackle the pressing energy, environmental, and climate challenges of our time.
Broadly, our research falls under two themes: Functional Materials and Collaborative Intelligence.
Functional Materials
Revolutionary technologies often owe their success to new materials. From doped silicon sparking the era of transistors and modern electronics to the Li-ion batteries powering today's electric vehicles, materials play a pivotal role.
Our research group focuses on designing functional material, encompassing solution synthesis and understanding structure-property relationships through laboratory and synchrotron characterization. We have a particular interest in hybrid organic-inorganic materials. What excites us is their immense design freedom in chemistry, stoichiometry, geometry, and topology. We navigate through these complex design spaces leveraging lab automation and data science toolkits, aiming to unlock their boundless functional potential.
Materials
Crafting an optimal energy device, such as a high-density battery cell, is like orchestrating a symphony. It is not just about having various top-notch materials (akin to skilled musicians) but ensuring they harmoniously work together: anodes (strings), cathodes (woodwinds), electrolytes (brass), separators (keyboards), and current collectors (percussion) - all in one.
Our research ensemble is on a quest to master this symphony of materials. We are interested in designing heterostructures, microstructures, and interfaces, focusing on understanding how operational conditions and user behaviors influence system performances.
We develop experimental tools to track materials’ spatial-temporal behavior and computational methods to diagnose their failure mechanisms. Our overarching goal is to reduce the time & costs of bringing new materials to energy conversion, storage devices, and clean technology deployment.
Energy
Collaborative Intelligence
Today, robots can help us in many ways. Some excel in tasks we can do but more efficiently, like robot vacuums cleaning floors at home, while others tackle challenges beyond our capabilities, such as the Mars rovers exploring another planet. However, the development of robot scientists is still in its infancy. A central challenge lies in teaching robots to make informed scientific decisions.
Our research group is interested in designing and building autonomous systems for scientific discoveries. We are interested in designing artificial intelligence agents that handle synthesis tasks, optimize processes, and generate experimental protocols. These agents seamlessly integrate with laboratory mechanics to execute their tasks.
A main focus of our team is understanding how robots can augment human capabilities in scientific research, rather than replacing them. We aim to cultivate an ecosystem where human intuition and artificial intelligence work hand-in-hand in materials design, driving collaborative innovation.
Autonomy
The transformation of measurements into structured information, followed by the extraction of scientific insights, has been the foundational scientific learning process for centuries. Advanced machine learning techniques offer the potential to accelerate this process, integrating high-throughput experiments with data-driven models in pursuit of knowledge generation.
Our research group is interested in designing digital representations of real-life measurements and developing predictive models correlating high-dimensional variables with scientific observations. A particular area of focus for us is the development of informatics tools to enhance the durability of perovskite solar cells, batteries, and fuel cell modules. We welcome collaborations in this domain.
Central to our methodology is the emphasis on the explainability of data-driven methods and human understanding. We prioritize interpretable machine-learning techniques and strive to extrapolate our findings from one energy system to another.